Table Of Content
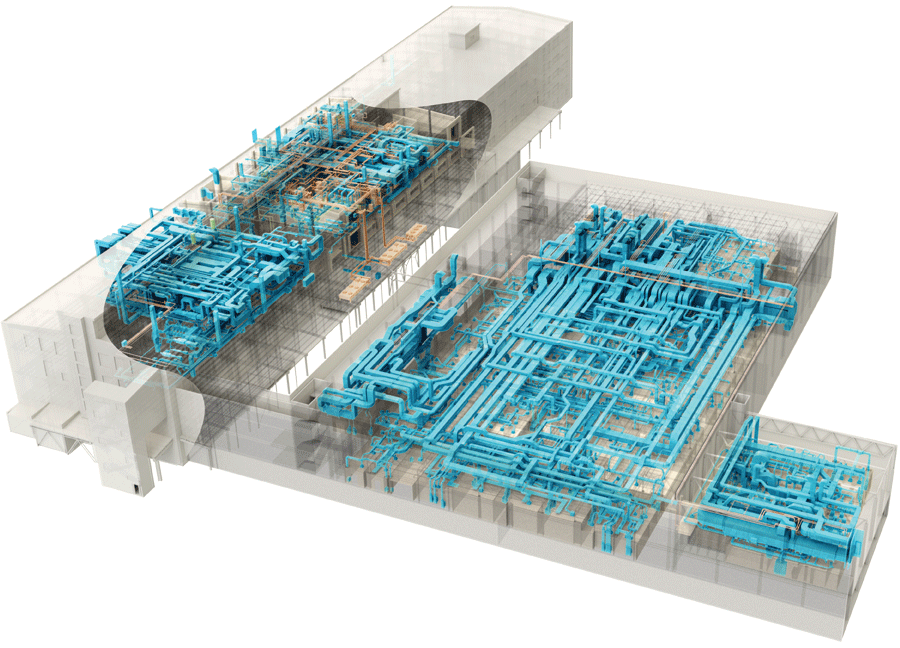
In this case, the test specimens would be considered a source of nuisance variability. If we conduct this as a blocked experiment, we would assign all four tips to the same test specimen, randomly assigned to be tested on a different location on the specimen. Since each treatment occurs once in each block, the number of test specimens is the number of replicates. When we have a single blocking factor available for our experiment we will try to utilize a randomized complete block design (RCBD). We also consider extensions when more than a single blocking factor exists which takes us to Latin Squares and their generalizations. When we can utilize these ideal designs, which have nice simple structure, the analysis is still very simple, and the designs are quite efficient in terms of power and reducing the error variation.
Crossover Design Balanced for Carryover Effects
Consider a scenario where we want to test various subjects with different treatments. Here are the main steps you need to take in order to implement blocking in your experimental design. First the individual observational units are split into blocks of observational units that have similar values for the key variables that you want to balance over. After that, the observational units from each block are evenly allocated into treatment groups in a way such that each treatment group is allocated similar numbers of observational units from each block. Suppose that skin cancer researchers want to test three different sunscreens. They coat two different sunscreens on the upper sides of the hands of a test person.
Error
Here is a plot of the least squares means for Yield with the missing data, not very different. There are 23 degrees of freedom total here so this is based on the full set of 24 observations. Generally the unexplained error in the model will be larger, and therefore the test of the treatment effect less powerful. Then, under the null hypothesis of no treatment effect, the ratio of the mean square for treatments to the error mean square is an F statistic that is used to test the hypothesis of equal treatment means. Randomized block ANOVA shares all assumptions of regular ANOVA. There are two additional assumptions unique to randomized block ANOVA.
Statistical Analysis of the Latin Square Design
However because our irrigation system can’t be fine-tuned, we have plots with different irrigation levels and within each plot we have perhaps four subplots that have the fertilizer treatment. To summarize, Irrigation treatments were randomly assigned to plots, and fertilizer treatments were randomly assigned to sub-plots. If the number of times treatments occur together within a block is equal across the design for all pairs of treatments then we call this a balanced incomplete block design (BIBD). Since \(\lambda\) is not an integer there does not exist a balanced incomplete block design for this experiment. Seeing as how the block size in this case is fixed, we can achieve a balanced complete block design by adding more replicates so that \(\lambda\) equals at least 1.
Finally, we walk through the steps that you need to take in order to implement blocking in your own experimental design. This type of experimental design is also used in medical trials where people with similar characteristics are in each block. This may be people who weigh about the same, are of the same sex, same age, or whatever factor is deemed important for that particular experiment. So generally, what you want is for people within each of the blocks to be similar to one another. Randomized block designs are often applied in agricultural settings.

Situations where you should use a Latin Square are where you have a single treatment factor and you have two blocking or nuisance factors to consider, which can have the same number of levels as the treatment factor. An alternate way of summarizing the design trials would be to use a 4x3 matrix whose 4 rows are the levels of the treatment X1 and whose columns are the 3 levels of the blocking variable X2. The cells in the matrix have indices that match the X1, X2 combinations above. At a high level, blocking is used when you are designing a randomized experiment to determine how one or more treatments affect a given outcome.
2 Split-plot designs
My guess is that they all started the experiment at the same time - in this case, the first model would have been appropriate. In this Latin Square we have each treatment occurring in each period. Even though Latin Square guarantees that treatment A occurs once in the first, second and third period, we don't have all sequences represented. It is important to have all sequences represented when doing clinical trials with drugs. This situation can be represented as a set of 5, 2 × 2 Latin squares.
This means the effect of cell phone use treatment (yes vs. no) on the dependent variable, driving ability, should not be influenced by the level of driving experience (seasoned, intermediate, inexperienced). In other words, the impact of cell phone use treatment (yes vs. no) on the dependent variable should be similar regardless of the level of driving experience. If this assumption is violated, randomized block ANOVA should not performed. One possible alternative is to treat it like a factorial ANOVA where the independent variables are allowed to interact with each other. To address nuisance variables, researchers can employ different methods such as blocking or randomization.
Next we can do the appropriate analysis for the fertilizer, recognizing that all the p-values for the plot effects are nonsense and should be ignored. In this case we see that we have insufficient evidence to conclude that the observed difference between the Irrigation levels could not be due to random chance. Minitab’s General Linear Command handles random factors appropriately as long as you are careful to select which factors are fixed and which are random.
NBA In-Season Tournament championship: Lakers defeat Pacers to win inaugural NBA Cup - CBS Sports
NBA In-Season Tournament championship: Lakers defeat Pacers to win inaugural NBA Cup.
Posted: Sat, 09 Dec 2023 08:00:00 GMT [source]
Here we have two pairs occurring together 2 times and the other four pairs occurring together 0 times. Therefore, this is not a balanced incomplete block design (BIBD). What we now realize is that two blocking factors is not enough! It looks like day of the week could affect the treatments and introduce bias into the treatment effects, since not all treatments occur on Monday. We want a design with 3 blocking factors; machine, operator, and day of the week.
Note that blocking is a special way to design an experiment, or a special“flavor” of randomization. Blocking can also be understood as replicating an experimenton multiple sets, e.g., different locations, of homogeneous experimental units,e.g., plots of land at an individual location. The experimental units shouldbe as similar as possible within the same block, but can be very differentbetween different blocks. This design allows us to fully remove thebetween-block variability, e.g., variability between different locations, fromthe response because it can be explained by the block factor. In that sense, blocking is a so-calledvariance reduction technique.
11 Remarkable GivingTuesday Stats for 2023 - The Giving Block
11 Remarkable GivingTuesday Stats for 2023.
Posted: Fri, 04 Aug 2023 07:00:00 GMT [source]
The sequential sums of squares (Seq SS) for block is not the same as the Adj SS. We could select the first three columns - let's see if this will work. Click the animation below to see whether using the first three columns would give us combinations of treatments where treatment pairs are not repeated.
Let's take a look at how this is implemented in Minitab using GLM. Use the viewlet below to walk through an initial analysis of the data (cow_diets.mwx | cow_diets.csv) for this experiment with cow diets. The following crossover design, is based on two orthogonal Latin squares.
In this case, a block represents an experimental-wide restriction on randomization. In this example we wish to determine whether 4 different tips (the treatment factor) produce different (mean) hardness readings on a Rockwell hardness tester. The treatment factor is the design of the tip for the machine that determines the hardness of metal.
In that situation, randomized block design can decreases the statistical power and thus be worse than a simple single-factor between-subjects randomized design. Again, your best bet on finding an optimal number of blocks is from theoretical and/or empirical evidences. In randomized block design, the control technique is done through the design itself. First the researchers need to identify a potential control variable that most likely has an effect on the dependent variable.
Randomized block design still uses ANOVA analysis, called randomized block ANOVA. When participants are placed into a block, we anticipate them to be homogeneous on the control variable, or the blocking variable. In other words, there should be less variability within each block on the control variable, compared to the variability in the entire sample if there were no control variable. Less within-block variability reduces the error term and makes estimate of the treatment effect more robust or efficient, compared to without the blocking variable.
No comments:
Post a Comment